Natesan, Nandakumar, Palka & Shrestha (2021)
Visual analysis has been a common tool used to analyse single-case experimental design (SCED) data. However, visual analysis is limited because it cannot detect subtle effects or control for trends and patterns in the data that may interfere with the interpretation of the data. As a result, there is growing interest in robust statistical methodologies to analyse SCED. The statistical approach selected must account for the unique characteristics of SCED data such as autocorrelation. This means that “traditional” statistical techniques, such as the t-test, are not suitable for analysing this type of data. This paper outlined specific advantages of Bayesian methods in the analysis of SCEDs and compared Bayesian Unknown Change-Point Models and Simulation Modelling Analysis for assessing intervention effects in SCED data. Bayesian Unknown Change-Point Models and Simulation Modelling Analysis are both simulation-driven approaches for analysing SCED data. The authors found that the estimates from Simulation Modelling Analysis did not meet published standards for establishing intervention effects (Kratochwill et al, 2013) in relation to two aspects – estimating effect sizes that account for autocorrelation and immediacy of the intervention effect, whereas Bayesian Unknown Change-Point Models did. The authors echoed the recommendations made by many others that statistical analysis and visual analysis should be used in combination for the transparent, replicable and objective analysis of SCEDs.
Click here to view the full article.
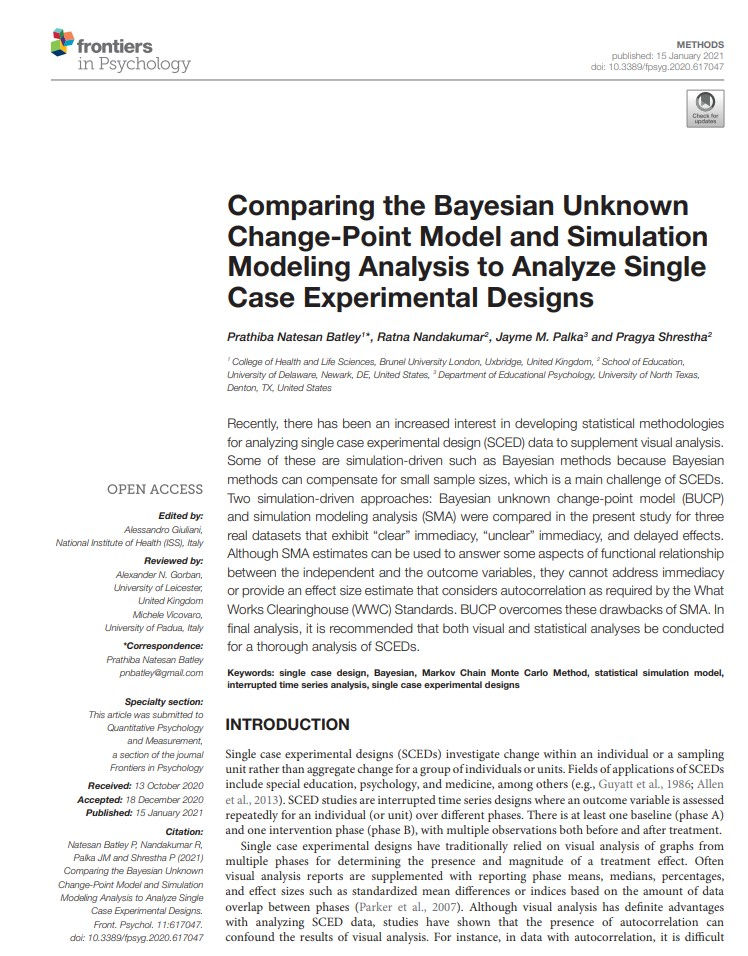
Full citation: Natesan Batley, P., Nandakumar, R., Palka, J. M., & Shrestha, P. (2021). Comparing the Bayesian unknown change-point model and simulation modeling analysis to analyze single case experimental designs. Frontiers in Psychology, 11, 617047.
Comments