N-of-1 Trials are Valuable Scientific Methods for Personalised Medicine
- ICN
- Nov 30, 2020
- 4 min read
Updated: Apr 1, 2024
Authors: Suzanne McDonald, James McGree & Jane Nikles

We were interested to see the reference to N-of-1 trials in the article by Wilkinson et al. (2020) ‘Time to reality check the promises of machine learning-powered precision medicine’ published recently in the journal The Lancet Digital Health. The authors endorsed the use of N-of-1 trials as a pragmatic method for understanding whether treatments work for individuals - the “goal” of personalised medicine. N-of-1 trials are one of the few scientific methods that provide information about treatment response at the individual-patient level. In N-of-1 trials, patients receive the intervention and control. Patients receive repeated treatment administrations over time. This more adequately addresses possible within-individual variability in response, which is not captured in designs that rely on few outcome measurements (Senn, 2018).
Applicability of N-of-1 trials
Wilkinson et al. state that N-of-1 trials “are only plausible in specific circumstances (eg, where the symptoms and participant circumstances are stable over time and the treatments have no long-term effects)". Indeed, N-of-1 trials are inherently best suited to the following contexts: (1) the condition is stable/chronic, (2) treatments have varying effects across patients, (3) treatments have a rapid effect onset and offset and are not curative, and (4) treatments require minimal “washout” periods (Nikles, 2015). However, symptomatic treatment for chronic conditions is very common in medicine and, to date, N-of-1 trials have been applied to many diverse areas including neuropsychiatry, musculoskeletal medicine, pulmonology, gastroenterology, psychology, cardiovascular, allergy and more. Therefore, we believe that N-of-1 trials have broader applicability than what is generally considered.
The appeal of N-of-1 trials
N-of-1 trials offer opportunities beyond determining individual treatment effects. They can be used to compare side effect profiles for different treatments, to de-prescribe current treatments that do not show clinical benefits, to compare different doses/combinations of treatments, and to investigate what is important to the patient by incorporating patient-centred outcomes into their design. Data from several N-of-1 studies with the same protocol can be statistically aggregated to provide information about individual- and group-level treatment effects. Aggregated N-of-1 trials can generate evidence of equivalent strength to parallel group randomised controlled trials and crossover trials with smaller sample sizes due to the statistical power yielded from repeated observations from each individual (Blackston, Chapple, McGree, McDonald & Nikles, 2019).
N-of-1 trials for estimating sub-groups
Wilkinson et al. believe "a more pragmatic route towards greater personalisation might be to shift the aim towards stratified medicine (ie, identifying and predicting subgroups with a better and worse response)". We would like to suggest that this could potentially be addressed within an N-of-1 framework. If subgroups were suggested a priori, aggregated N-of-1 studies could be powered to estimate differences between subgroups. This could be achieved by including a covariate into the linear predictor of the model, and assessing the evidence that the effect is different from 0. For example, if gender is thought to be a subgroup and an effect size is supposed, then a power analysis could be conducted to determine what sample size is needed to determine this effect is significant. We would then need to recruit a minimum number of females/males in the trial. This highlights that N-of-1 trials can estimate individual and population treatment effects, as well as to explore, identify and estimate subgroups.
Designing N-of-1 trials
N-of-1 trials are not a panacea and designing them requires careful consideration to avoid methodological issues that can weaken internal validity. For example, decisions about the length of the periods in the N-of-1 trial need to be made with care in order to account for potential carryover effects and the immediacy or duration of the treatment effect (McDonald, McGree & Bazzano, 2019). The International Collaborative Network for N-of-1 Clinical Trials and Single-Case Designs (ICN) is a global network of clinicians, researchers and healthcare consumers interested in these designs. The ICN offers expert advice and assistance to researchers and clinicians to help design high-quality, scientifically rigorous N-of-1 trials.
We were delighted to see support for the use of N-of-1 trials in personalised medicine. It is our mission to continue to promote and advocate for wider recognition of their value in numerous suitable contexts.
About the authors
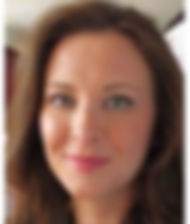
Suzanne is co-chair of the ICN and a Clinical Trial Coordinator at the Centre for Clinical Research at The University of Queensland, Brisbane, Australia. Email: suzanne.mcdonald@uq.edu.au
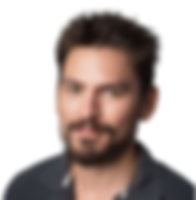
James is the Statistics theme leader for the ICN and a Professor at the School of Mathematical Sciences at Queensland University of Technology, Brisbane, Australia. Email: james.mcregree@qut.edu.au
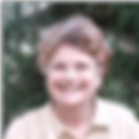
Jane is co-chair of the ICN and a Principal Research Fellow at the Centre for Clinical Research at The University of Queensland, Brisbane, Australia. Email: catherine.nikles@uq.edu.au
References
Blackston, J.W., Chapple, A.G., McGree, J.M., McDonald, S., Nikles, J. (2019). Comparison of aggregated N-of-1 trials with parallel and crossover randomized controlled trials using simulation studies. Healthcare, 7, 137.
McDonald, S., McGree, J., Bazzano, L. (2019). Finding benefit in n-of-1 trials. JAMA Internal Medicine, 1, 454-5.
Nikles, J. (2015). N-of-1 What are N-of-1 Trials. In J. Nikles & G. Mitchell (Eds.) The Essential Guide to N-of-1 Trials in Health. SpringerLink.
Senn, S. (2018). Statistical pitfalls of personalized medicine. Nature, 563, 619–62
Wilkinson, J., Arnold, K.F., Murray, E.J., van Smeden, M., Carr, K., Sippy, R., de Kamps, M., Beam, A., Konigorski, S., Lippert, C., Gilthorpe, M.S. (2020). Time to reality check the promises of machine learning-powered precision medicine. The Lancet Digital Health. (Online first).